Data-based decision-making systems are increasingly affecting people’s lives. Such systems decide whose credit loan application is approved, who is invited for a job interview and who is accepted into university. This raises the difficult question of how to design these systems, so that they are compatible with fairness and justice norms. Clearly, this is not simply a technical question – the design of such systems requires an understanding of the social context of these applications and requires us to think about philosophical questions.
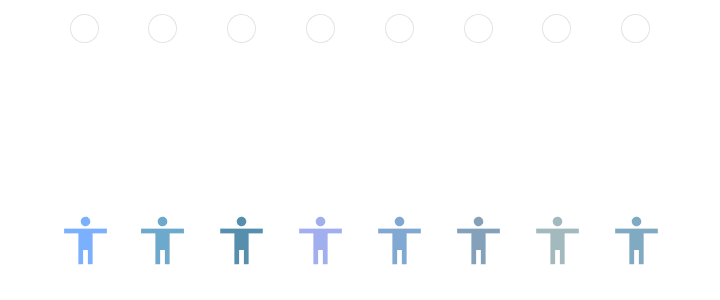
Counterfactuals in XAI
Counterfactuals in XAI
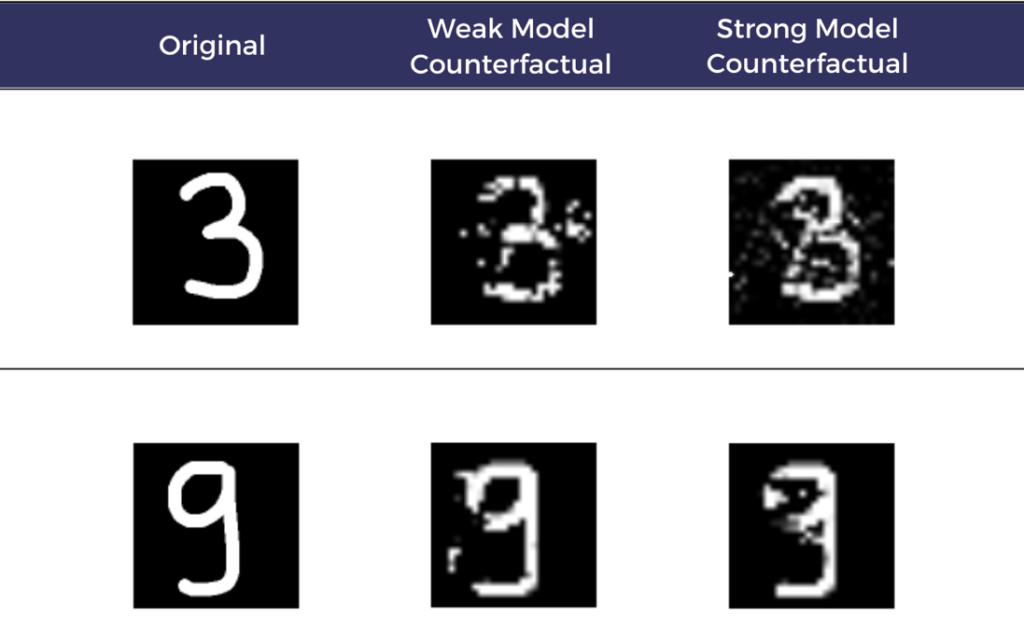
Research Scope
The scope of this research is to reduce the bias toward a specific feature or a set of features in the training dataset to increase the model fairness without altering the correlation value between the rest of the features on the outcome. The paper focuses on measuring the quality of the generated counterfactuals, their impact on the feature importance / corre- lation, and increasing the model’s fairness with each batch of generated counterfactuals.
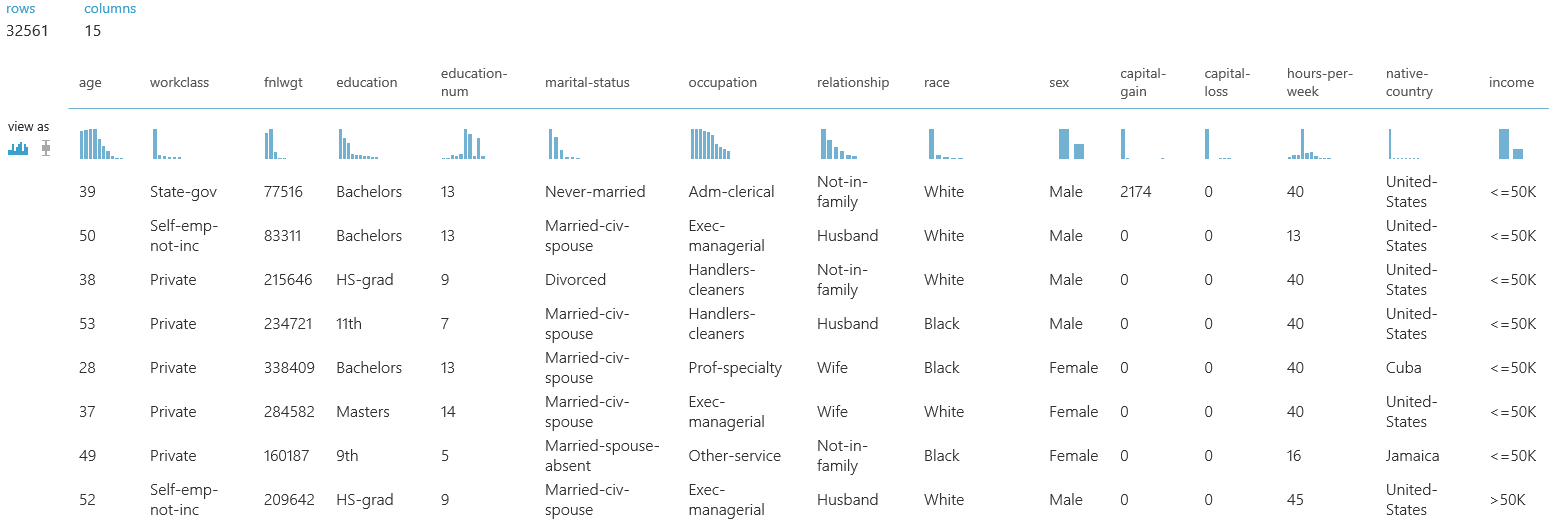
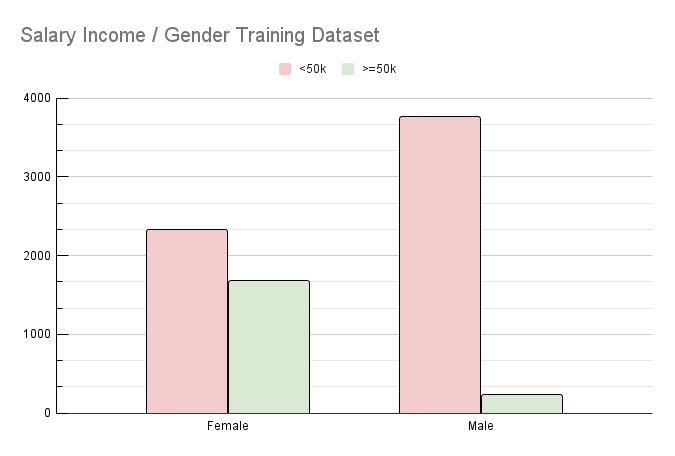
Sample Counterfactual
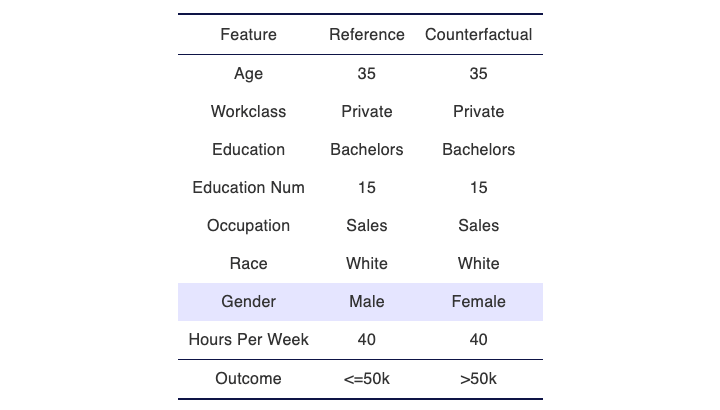
Results
The counterfactuals were generated using seven iterations, each consisting of a batch of 1,000 samples for measurements and counterfactuals. The decrease of accepted solutions with each iteration results from an increase in model robustness and a proof that the generating algorithm finds fewer solutions or the found solutions have too many changes to be accepted. To evaluate the model fairness update, a benchmark dataset was used after each new batch of synthetic data was pushed back into the training dataset, and the model was updated. After each benchmark, we extracted the number of times each feature was changed for both generated and accepted solutions. For the initial iteration, Gender was the primary feature that was changed to generate a potential solution (696 potential solutions, 302 passing the threshold). As the model is updated and the fairness is corrected, later iterations observed a decrease in solutions with changes in Gender and mainly focused on other, more relevant to the real-world features, such as Age, Education Num and Hours per Week.
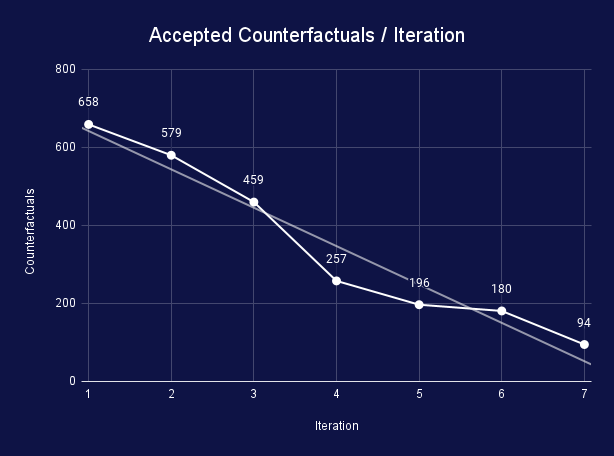
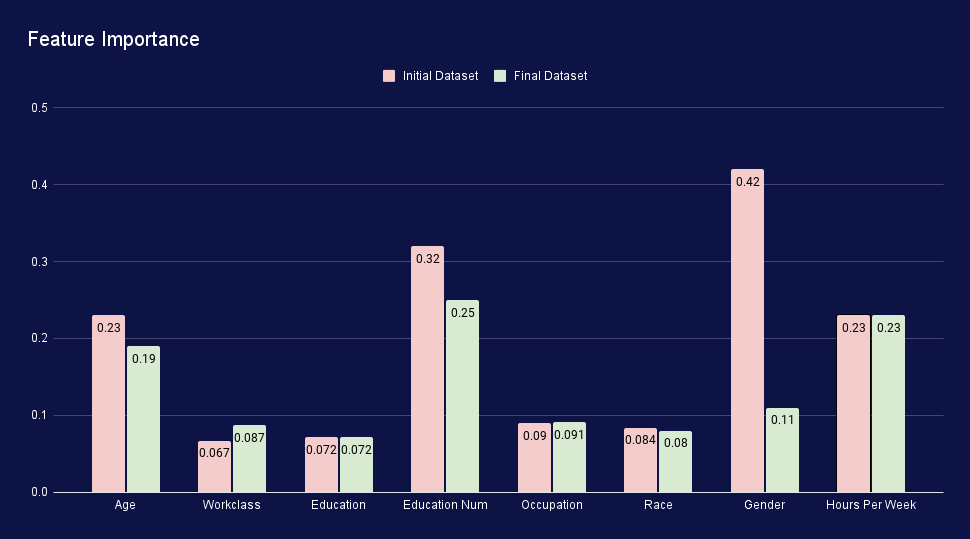